Reshape your business with data-enabled organisation
Over the years, I feel very fortunate to have constant access to many challenging digital transformation projects. After leaving Alibaba, I was involved in the Big Data advisory Group for Beijing Municipal Government, the write-up of the Big Data Research Report for the crossborder data of Guangdong-Hong Kong-Macao Greater Bay Area, and the establishment of Data Platform for JD Digital, etc. At the same time, I was intensively engaged in the digital transformation process of Sequoia Capital’s invested companies.
My teaching work at Tsinghua University and the University of Hong Kong has given me many opportunities to exchange views with the leadership of the Internet and financial institutions. I am indebted to all of them for their trust and benefit.
For a proper digital transformation, the corporation must first understand “Big Data” and not think of it as another version of data resources but game changer in revolutionizing the way company make decision and manage the business. In starting to write this book, apart from hoping to summarise past experience, i also want to share my insight on the step to build a “data-enabled organisation” as a sustainable growth framework under digital transformation to senior management.
Essentially, the value of Big Data comes from the continuous reinvention and discovery of data collection to usage in the reinforcing loop ( between data application and data accumulation) of business operation. The recent study of data network effect (Clough & Wu 2022) had provide an important insight, that more users get you more data which lets you build better insight and ultimately a better product (or service) to get more users. It is also known as “data flywheel”. The key conditions is that learning from one user can be translate into a better product for other user and such enhancement happen to benefit the current user of the product within the same life time. For example, the more that Taobao (Alibaba’s C2C marketplace) learns about buyer behaviour and the recommendation engine they conduct, the more it can personalise the experience, making the marketplace more valuable to everyone. However, it is pretty that company undergoing digital transformation has paid little attention in data network effect.
Figure i illutrate how this positive self-reinforcing user expectations play a role in single data flywheel(See Figure. i). However, in a broad sense, Big Data is harnessed as “THE” super resource for future business or product development. Through symbiotic processes across multiple data flywheel, the stacking effect can supercharge data resources to create immeasurable value, which is why Big Data has been hailed as the diamond mineral of the future.
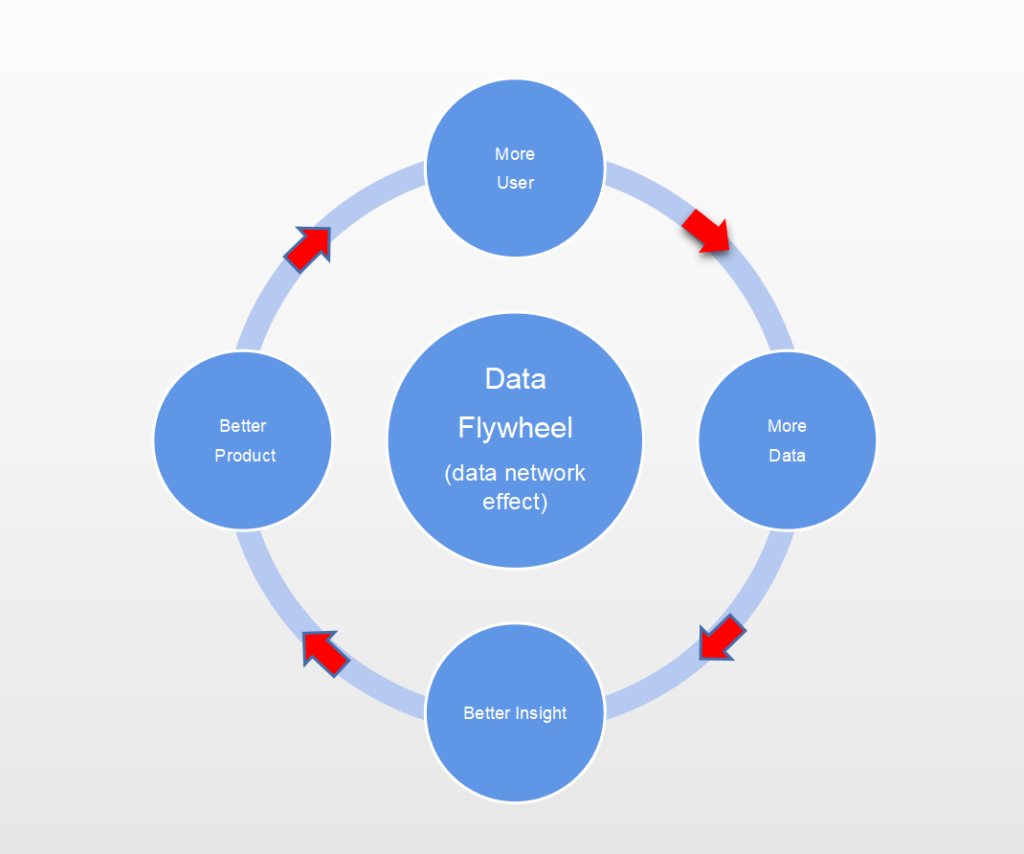
As I pointed out in my first book, “The Business Revolution of Big Data”, published in 2014, data considered redundant in one scenario can become a treasure when integrated under another scenario. Data network effect only arise to the extent that the learning is across users. In my second book, “The Nature of Big Data”, I reiterated that Big Data is formed through constant connectivity. In weaving this “Data network effect”, we need to learn to build ongoing mutually beneficial relationships.
It is undoubtedly a long journey for corporates to effectively use data, but it is always worth pursuing. As long as business objectives are clear, it is easy to measure how well they perform. However, it is not the same with “data accumulation”. If a corporate lacks a clear strategic direction, it will not only fail to cultivate good data but will also cause a substantial internal drain on resources, such as loss of confidence of management and general employees in terms of digital transformation. In fact big data usually refer as large and complex data resources that require cost-effective management before extraction of insights from them. However the confluence of data resources, advanced AI, super computing power with sound data strategy have open the new possibilities for many innovation ahead.
As mentioned earlier, the essence and value of Big Data come from the connection between data resources across multiple data flywheel. Still, the broader the scope for data governance, the more difficult it is to guarantee its quantity and quality at the same time. Moreover, the more diversify in nature of the data resources collected, require the more resources to fix the disparities (out of context).
In my view, the effective leverage of simple data network effect in digital transformation exists within a reasonable scope of data that meets the current strategic objectives. If the strategic goals are set appropriately, then success is already in sight, but getting the data to circulate fully through the multiple data flywheel will involve organisational structure changes (both breaking and establishing) and a change in the mindset of the business, technology, resource allocation and talent development will be at the core of the transformation.
Herbert Chia's "The Big Data" and "The Nature of Big Data" are both best-selling books in the Big Data category. The former is a masterpiece that fully demonstrates how to build a data mindset in the digital era; the latter focuses on the second half of Big Data on its value and unlimited possibilities. The author believes that whoever can understand the essence of data will get out of the maze and become the winner! Both books have been published in simplified Chinese by Cheers Publishing. --Editor's note
It can be seen that investing too many technical resources or human costs directly before building a top-level framework (data strategy) can easily lead to internal conflicts and disputes within the enterprise before the techonology has achieved results. This is the main reason why few enterprises suceed in digitalisation locally and internationally.
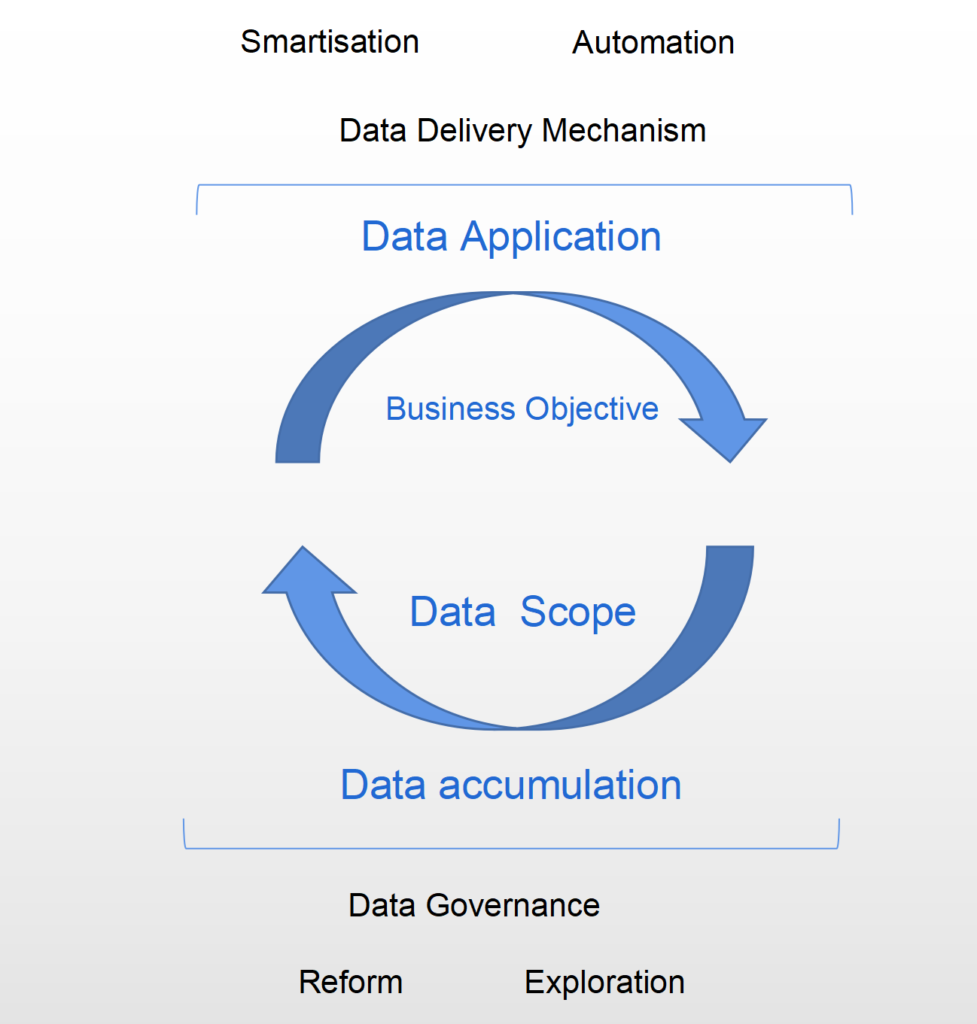
As mentioned earlier, the core of digital transformation is the “application” and “accumulation” of big data resources (See Figure ii), especially in today’s rapidly changing world, where agility has become the key to survival and innovation. Agility needs to be backed up by a robust data capability. As a result, many companies are becoming more eager to digitally transform themselves. However, many business leaders, before going all out with their digital transformation, have the following common issues to consider:
How to set priorities in the transformation? How should I choose the right entry points? How do I balance data security and personal privacy with business development? How do I find the right CDO? How can I tell if I’ve done the transformation right?
It seems everyone realises that taking a wrong turn can cost you dearly!
Management’s hesitation may come from the three levels of misconceptions enterprises face during the digital transformation process, and enterprises can save a lot of wrong turns if they can identify these misconceptions early.
First of all, when it comes to digital transformation, the focus of most companies is mainly on improving efficiency. When it comes to implementation, they often fall into the first level of misconception; they think that the business units in the company can simply or only use the data they already have and deploy analytical tools and algorithms to solve the problems.
This way of thinking lacks an external perspective and does not recognise that the value of Big Data lies in the insights that can be gained by connecting it to external data. This misconception leads to each business unit minding their own turf within the organisation. Without coordination, data resources are fragmented and lack synergy and a necessary layout for strategic data resources. Let’s not forget that what sets Big Data apart from ordinary data is its ability to achieve data blending within or outside the company.
In the second level of misconception, although companies are aware that the speed and effectiveness of digital transformation are determined by continuous reinvention and discovery of data collection to usage in the reinforcing loop (data application and data accumulation) of business operation. they may have overlooked that solving this problem causes the butterfly effect. It is a matter that involves not only internal interests and external competition but also the distribution of benefits and parochialism, and organisational dissonance is often the case. Therefore, digital transformation also involves higher-level organisational issues, which are aspects of traditional management thinking that need to be changed.
The third level of misconception is the balance between “planning before moving” and “small steps”. Large companies tend to get stuck in a dormant state due to over-planning; while small steps are good for rapid iteration, they lack a holistic approach, resulting in repetition, redundancy and eventually overturned work.
Only a few companies have this understanding: to consider trial and error as part of their strategy and top-level framework, constantly review and rectify during implementation, learn from their failures, and find effective solutions. In short, a successful digital transformation strategy for innovative companies is not thought out of the blue but rather something that is done and fought.
All companies should be aware of these issues before starting their digital transformation. Of course, there is no perfect answer to these questions, and the key is not to let the cost of trial and error be too big. If I had to put into 2 points the lessons I have learnt in my ten years of experience, I would say: firstly, don’t be too ambitious and get carried away; secondly, don’t see digital transformation as pure technical play.
Promoting data network effect or data-enable learning capability within a digital transformed organisation is, in essence, a set of methodological practices i refer in this book as “data reinvention framework”.
The Big Data industry has been developing for more than ten years now, but there is undeniable that many corporates are still in the stage of “consoling themselves with false hopes”. Data is closely related to data-driven capabilities as a critical factor of production in the digital economy, and some corporates even refer to this newfound capability as “data power”.
As one of the pioneers in the Big Data industry, I have been committed to promoting the popularisation and application of data-driven thinking. From my book “The Business Revolution of Big Data” to “The Nature of Big Data”, I am trying to make enterprises feel the importance of data network effect through these publications.
A predecessor once said to me, which I have taken to heart over the years, “the premise of using Big Data well is first to assume that all data can be collected”. Jack Ma also once said that true Big Data emerges when you haven’t figured out how to use that data yet.
These two statements illustrate the importance of not letting thoughts limit possibilities as if to say that more data is better, but in digital transformation, with limited resources, the execution path is equally important, and the key to success is to focus. Therefore, a data strategy is critical, as many corporates are easily overwhelmed by the lack of a defined data scope, fearing that there is not enough data, while at the same time, concerned about resources wastage.
It is not easy for corporates to determine whether their data resources are sufficient for future business needs in the present, so it is crucial to take stock of the current state of data (Maturity of data from prospective to actual).
Depending on the data scope, corporates can plan for the allocation of resources, organisational structure, security mechanisms and safety compliance, etc., in the cycle of “data application” and “data accumulation” (see Figure ii).
To deepen the reader’s understanding, I have introduced the six modules in a data life cycle with the six key governance elements into a 6 x 6 matrix ( in Chapter 5 & 6) so that corporates in different states can find what suits them better and the right entry point.
I’ve always believed that it’s essential to understand these matters before discussing the technology issues such as Data Plaform(in Chapter 8); otherwise, it is easy to fall into the myth of technology. About the set of methodological practices on Big Data, starting with my first book, ten years of Big Data’s time goes by, and ending with my latest work “Data Reinvention”, should bring my work on driving the business value of Big Data to a close, but open a new chapter on how to use Big Data morally and ethically.
I want to thank the leaders and senior management of Alibaba, JD, WeBank, TalkingData, Yuntu Technology, Didi, Meituan, Vipshop, Li & Fung, China Construction Bank, Bank of China (Hong Kong), Bank of East Asia for their support to me and help with this book.
My appreciation to my four assistants, Wang Xiuqing, Zhang Honghai, and Lu Boqing, maple Kwong for their efforts in contributing to this book over the past two years.
Last but not least, my heartful gratitude to my wife Doris and my two daughters for bearing with me from hiding in my writing and not doing my chores.