Whoever is first to use the data reinvention will be first to reap the dividends of digital transformation
Today’s world is full of uncertainty, and “black swan” events are far beyond human ability to avoid, but access to data power can help you deal with an uncertain future.
The widespread use of Big Data and the resulting changes in economic activity has generated global interest. For example, with the advent of the internet, humans have broken through the natural barriers of time and space. Today, more than half of the planet’s population already has access to the internet at a meagre cost. Over the decades, driven by the network effect, the internet has fully penetrated every aspect of social development, profoundly changing our production and way of life.
For example, although I live in Hong Kong, I can connect to cities like Beijing, Shanghai, and Guangzhou via the internet. At the same time, online communities that are aware of cutting-edge technology trends “reside” in large cities such as Boston and London.
Today, many aspects of urban life are located in different physical spaces and generate massive amounts of individual data spreading across shopping, social, entertainment, work, etc. These are stored separately and largely disconnected from each other. Each “silo” data is stored in the hands of a separate service provider, and the amount of data is immeasurable even for the users themselves. In short, data has become increasingly scattered.
In addition, with the spread of cloud computing and machine learning algorithms, artificial intelligence has become a central driver of economic development, taking data-drivenness to a whole new level. However, the key takeaway from this process is not the advancement of technology but the increase in innovation capability within organisations due to the change in data mindset.
If companies want to make the most of the dividends of the digital economy, they must first understand that one of the key features of the digital economy is the network effect. In a smart world where everything is connected, data is being generated and interconnected all the time to create new value. Data has a lifecycle, and its lifecycle has the following characteristics:
- There is a tendency for self-reinforcement in the relationship between data and intelligence.
- Data is becoming cheaper to store, but it is costly first to refine and govern it.
- The marginal cost of data, which can be reused in multiple scenarios, will eventually approach zero.
- Data is easily undervalued, as its value is primarily determined by how well it is used.
- The immediacy and integrity of data begin to decay the moment it is collected.
With the successive spread of technologies such as 5G, IoT, and blockchain, data-drivenness will profoundly impact all industries. Each new technology can affect the distribution pattern of data resources; for example, the home entertainment data captured by smart TVs is by no means accessible by smartphones; the emergence of blockchain has made data more decentralise and much trustworthy, etc.
Digital transformation is not all about technology
While we are all excited about artificial intelligence and Big Data development, have we ever wondered why so few companies are profiting from it? The answer is simple: most companies are still in the early stages of Big Data development. Don’t forget that companies like Google and Alibaba started their digital transformation over10 years ago.
Although we cannot yet describe the full picture of Big Data, the term’s connotations are still evolving. I remain convinced that the future is a fast-moving world whose lifeblood indeed depends on real-time decision-making and product design driven by massive amounts of data. Big Data will fundamentally revolutionise the way people live, and businesses operate and even give rise to new social forms and industries.
As externalities and network effects characterise Big Data, whoever can stay ahead of the curve in a data strategy will likely have a clear first-mover advantage. If they miss out, they will lose the opportunity and the competitive barrier for the future.
So what is the real state of play? Data from a 2018 survey of the executive community by NewVantage Partners reveals that more than 90% of executives at Fortune 1,000 companies say their companies are accelerating their efforts to increase their investment in Big Data and artificial intelligence and that a majority of executives see this shift as imminent, as the consensus is that companies are facing unprecedented challenges
However, over time, executives at many of these companies say they are not reaping the satisfactory rewards of these cutting-edge technologies. This fact is not as surprising as the proportion of companies reporting that they have transformed into data-driven businesses has declined. Even more surprising is that this proportion has continued to show a downward trend in recent years.
Executives of companies that failed to transform cited a lack of a strategic view of data and cultural challenges as the main reasons for their failure. At the same time, technology-related factors accounted for only 5%.
It seems to me that the root of the problem is not being understood, just as buzzwords such as “data facilitation platform”(DFP), “data governance”, and “smart city brain” seem to be true, but they are not yet exploring.
I have always been interested in how to accelerate digital transformation. Still, I want to start by saying that the digital transformation discussed in this book is relatively narrow in scope. I am looking more at how digitalisation and being data-driven can inspire innovation in the organisation.
Over the past few years, I have served as a consultant for many large organisations, and I have also done a lot of consulting on Big Data for the Beijing and Shanghai governments. During this process, a key issue emerged: in all these cases, the problems encountered by the organisations or institutions were similar, and some of them kept repeating.
Whether a corporate wants to use Big Data or AI to grow its business or a government wants to use AI to solve urban development problems, it seems that everyone is looking for technology solutions. The concepts that carry these dreams include “smart city brains”, “leadership dashboard “, “DFP”, etc.
From what I can see, most of these concepts have only found their “names” but not their “prescriptions”. So, even if you have a strong will for digital transformation, it is still futile without the proper practical solutions.
In my opinion, the first task of digital transformation is not to choose a technology tool but to identify a more explicit business logic, to define how to reach the inflexion point of development through digital transformation, and to explore new business patterns and models, and then to develop practical solutions around this goal.
Remember: the clearer the goal, the more focused the resources and efforts of digital transformation will be, and the better the tracking and iteration on results so that the cost of trial and error will be reduced and the business will be more agile.
On the road to digital transformation, apart from the business logic, never underestimate employees’ resistance towards new trends in technology. When employees (including management) believe that digital transformation may threaten their jobs, they resist the change differently. Leaders need to recognise this employee sentiment in time and, while stabilising it, tap into success stories to lead internal buy-in.
We can think of digital transformation in an organisation as a positive cycle: firstly, using data-driven capabilities to enhance the effectiveness and innovation of the organisation, based on a consensus among senior executives.
Secondly, in achieving the goal, we develop the data competence of all employees, build a culture that identifies with the company’s digital transformation, and then form a digital strategy and tactics. In this way, the starting point for digital transformation is to take stock of the organisation factors that facilitate data-driven initiatives: data resources and the talent pool.
Data will be an essential resource for businesses in the future and is inextricably linked to the development of artificial intelligence. Still, the fact is that not many companies truly understand the relationship between the current state of the business and the use of data resources, let alone identify the gaps between future data needs and the current ones.
It is fair to say that unused data is like rubbish in a warehouse, which is not valuable to the business but rather a costly drain.
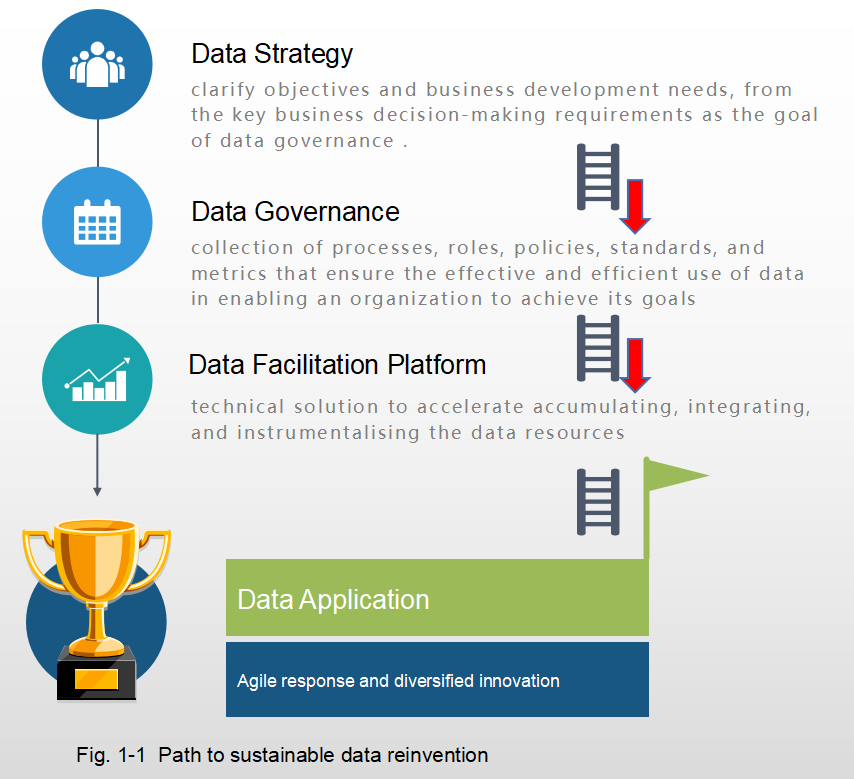
Data strategy, data governance and Data facilitation Platform(DFP), the path to sustainable data reinvention.
Digital transformation is not an easy task at all. In addition to technology, it is also important to follow a transformation framework, which has three key components: data strategy, data governance, and DFP.
The composition of Big Data is multi-source and heterogeneous, which contains four major trends: 1) Complexity nature of cross-border and cross-domain data compliance, 2) Mechanism to embrace data sharing, 3) Practice on a mixture of internal and external data for performance, 4) Data privacy and security become a major concern for data usage, which indirectly influence corporate data strategies while tied to the government policy development.
In the three stages of digital transformation, companies should follow the “8-step approach” to achieve breakthrough successfully, and I will elaborate on the details of this “8-step approach”.
I recommend that in the initial stages of digital transformation, corporates can start by defining the scope of their data strategy (Step 1) from the key business decision-making requirements and then use this as the goal of data governance (Step 2) and technology assurance (Step 3); and then gradually introducing machine learning algorithms to enhance the ability to leverage the massive amount of data (Step 4).
- Step 1: Focus on the decision-making process and strengthen data analysis capabilities.
- Step 2: Build data resources and develop data standards.
- Step 3: Develop management specifications and build a data governance platform.
- Step 4: Strengthen the ability of in-depth analysis on a massive amount of data
We need to pay attention to step 4 of the “8-step approach”: “Strengthen the ability to analyse massive amounts of data in depth”, which is a watershed for organisations to upgrade their data-driven capabilities and is also the way to acquire the ability to analyse corporate’s massive amount of data in depth. Big Data is a data lifecycle from being acquired to being stably used, which requires a set of sophisticated processes to manage to ensure the data’s quality, timeliness, and consistency.
Starting with Step 5, “Building a strategic reserve of external data”, the data ecology is already viewed from an external perspective, in which the borders of the data lifecycle become even more expansive.
In the process of digital transformation, primary data capability build-up, such as the improvement of data analysis and governance capabilities, the establishment of data standards and specifications, and the construction of data “warehouses” and back-end tools for data management, all need to be continuously strengthened with the addition of external data. After introducing external data, corporates should focus on the following four steps:
- Step 5: Build a strategic reserve of external data.
- Step 6: Build external innovation capabilities for data.
- Step 7: Promote the open-up and sharing of your data.
- Step 8: Optimise the strategic blueprint of the data industry.
The best time to introduce a DFP (Data facilitation Platform) as technical support is when the corporation can clearly understand the importance of data governance in the whole data lifecycle, which is why I wrote this book in the first place.
I believe that the essence of the DFP lies in accumulating, integrating, and instrumentalising the data resources that can be shared within the organisation, helping the corporate innovate flexibly and quickly to achieve its strategic goals.
Blocked, isolated, slow and leaky are the four primary hurdles to the implementation of data strategies
Although the logic of digital transformation does not seem difficult to most people, there are many practical problems during the actual transformation process, resulting in many corporates being squeezed out halfway through the process by the companies of the new era.
Through in-depth research, I believe that all the digital transformation predicaments of organisations can be described as “Blocked, isolated, slow and leaky”, and the “CXO“, management and even the lowest-level staff in the organisation should all feel it. These four predicaments reveal the inadequacy of data governance in the organisation, the lack of data governance capability and the lack of confidence of internal staff in digitalisation.
- Blocked: The huge amount of information in daily data and reports makes it difficult for decision-makers to capture valid information. For example, decision-makers have a backlog of daily, weekly and monthly reports, research reports and competitive market analysis reports on their desks. It is too difficult to identify and distil truly useful information from so many reports, so managers often lack useful data to support their decisions in their daily operations.
- Isolated: Information is scattered across different departments and is not effectively integrated. Data silos between business units are serious, and data analysis is fragmented, with little communication and integration. No one in the organisation understands how information or data is distributed and how to access it, so Big Data is just an empty talk.
CXO in this context refers to a set of senior management positions such as CEO (Chief Executive Officer), CFO (Chief Financial Officer) and COO (Chief Operating Officer) of an organisation. — Editor’s note
- Slow: There is a severe lag in the detection and handling of business variances. Many corporates lack proactive mechanisms and long-term monitoring mechanisms to deal with business changes. When business problems arise, managers are often kept in the dark. The lag in bottom-up information leads to a severe delay in solving problems, leading to greater difficulty in comprehensive analysis and parallel processing when dealing with multiple and complex issues.
- Leaky: The key analytics cannot be effective, with each department operating on its own. The whole process lacks proper management from problem identification, thematic analysis, and solution development to validation. Corporates continue to invest in resources, and the costs keep surging up. However, there is no insight and experience gained from data collection, collation, analysis, and evaluation and tracking, leading to ineffective data governance measures and resource wastage.
Once the above four hurdles to implementing data strategies are resolved, we can move on to more fully scale “data governance”.
With data governance, never use short-term solutions to solve systemic problems
As technologies such as unmanned driving, robotics and deep learning become more popular, the changes brought about by constant technological innovation has become a factor of uncertainty that many corporates cannot ignore. Therefore they are exploring the context of “data-drivenness”.
The first step is to use data to make accurate decisions and then deepen data decision-making capabilities into the business process. At this point, business innovation driven by data is born. This is where the process “data driving business transformation to business driving the technology” comes into play.
For example, Alibaba has derived Ali Finance from its Taobao business, JD Mall has incubated JD Digital Technology, and Tencent has led the establishment of WeBank, all of which are magnificent transformations that have caught everyone’s eyes. Therefore, I hope that you will not forget to look up at the sky when you are working diligently with your head down.
In digital transformation, the most common problem of most corporations is that they are too much in pursuit of short-term benefits while ignoring the traditional vertical division of labour and information management, which is detrimental to knowledge reuse, co-creation and accumulation. Corporations are prone to lose their innovative power as a result.
Therefore, I propose here the “3 tactics” for data governance in the digital transformation process.
- Make the development of data strategy a priority.
- Set the scope of data based on the strategic direction and, through data governance, solve the “aggregation – interconnection – application” issues throughout the data lifecycle.
- Establish the DFP as a corresponding technical platform rendering automation for efficiency improvement as the above 2 “tactics” mature.
As mentioned earlier, there are different approaches to data governance and technical requirements at various stages of data drivenness. For example, the analytical capability of the business is first and foremost about whether the setting of performance indicators is sufficiently sensitive and consistent. However, when dealing with large volumes of unstructured data, such as where data relating to customer complaints is subject to machine learning, it is crucial to retain the data in its original state as far as possible, as we want to both integrate customers’ complaints and retain the original content of the complaints and use it for other analytical purposes.
If an organisation has reached data capitalisation, then the data’s quality and security compliance become extremely important.
With many businesses wavering between data governance and business priorities and the market for innovation-driven businesses changing rapidly, the mismatch between data governance and data complexity can easily lead to a vicious cycle. The result is that not only do companies fail to get their long-term data governance in place, but they also drag down short-term business development.
Organisational structure and incentive mechanism are the prerequisites for good data governance and therefore, DFP.
Stephen Goldsmith, former Deputy Mayor of New York, once shared his experience of building a smart city with me. He said that it is inconceivable that a proven organisational structure that has been inherited for a century could be adapted at a stroke to the shocks of the new digital era. Until the key benefits arrive, we still have to proceed step by step.
No sound digital transformation can be complete without an agile organisational structure and incentives to break the mould. From my experience, the technical architecture is as important as the governance organisation, and in recent years many corporations and local governments have set up data management committees. The core concept of data transformation is to use organisational synergies to promote “interconnection propelled by application and application propelled by interconnection”: the “interconnection” here is not only about the connection between data, but also about the connection between people-oriented organisational structures, both vertically (between business units) and horizontally (between functions units).
Goldsmith’s book, “The Responsive City: Engaging Communities Through Data-Smart Governance”, intended to explain how to create a smart city with fast response, data sharing and efficient services in 6 major areas: smart citizens, smart environment, smart economy, smart talents, smart government and smart concepts. The simplified Chinese version was curated by Zhanlu and published by Zhejiang People’s Publishing House in 2019. –editor’s note
In essence, the DFP is about strengthening the ability of the back office (sometimes addressed as the middle layer platform in China) to channel data resources to support innovation and enhance the efficiency of governance and operations through technology to achieve effective use of data.
Overall, the primary function of the DFP is to enhance the aggregation, reuse, sharing, co-creation and rapid development of data within the designated scope of the data strategy. To implement a data strategy and better data governance, you can build a DFP.
Sharing and co-creation are the pinnacle value of a DFP.
The primary purpose of a DFP is not to create data silos. On the contrary, it is only when the organisation’s system and culture have reached the “break” and “make” stage in operating a DFP that it has the necessary conditions to talk about data sharing on a large scale.
From Alibaba to the companies and cities I have worked with as a consultant, they all have practical incentive mechanisms in place. “Monthly data billing” is a great tool to let everyone know how data is being supplied and demanded between departments and can fully promote internal understanding of the core concept of the DFP (application propelled by interconnection).
I remember one year, in Alibaba’s annual meeting of presidents, Jack Ma said that none of the presidents reached the target that year because everyone was not contributing data well. Therefore, the nurturing of the concept and culture of data sharing mindset reflects that a good data governance and DFP not only can efficiently support the existing business but also perform its greater role: when the innovative business incubated by the organisation has a DFP, each department can build its data platform while linking with other departments’ business data. Even if the existing DFP is not 100% compatible with the business, its structured, high-quality data resources and robust data services function may be good enough to support the rapid emergence of new businesses.
The true value of the DFP can only be brought into play when everyone learns to give up the ego and dedicate themselves to the greater good and reasonably frontload data resources onto the DFP to achieve “co-creation”. Realising the embodiment of the virtue, “every stream eventually meets at the ocean” (embracing for the greater good).