Agile transformation is driven by a urgent need to respond quickly to new opportunities, react to disruptions and find way to be more efficient in today’s business.
The Big Data industry has been developing for ten years, and so has my bonding with Big Data. As a new factor of production in the digital economy, data is closely related to its ability to drive business; I hope readers will be able to understand the following truth: Big Data is not far away, and it is rapidly and profoundly changing our society, environment, economy, and even our everyday lives. The definition of big data is yet to define with evolution of new techonology such as strong AI and metaverse.
Over the last few years, I’ve been serving as a consultant to government agencies and many corporates to help them implement digital transformation projects. With a data thinking mindset, I have exalted my experience into a practical methodology. I want to share my experience humbly and, at the same time, draw inspiration from those with common interests.
I hope everyone living in this era will have a more practical understanding of implementing big data and succeed in digital transformation for our cities and corporates to build a better and sustainable world.
【Big data as a new way of thinking 】
Big data, a new frontier for every country and enterprises
Increasing digitalisation brings huge amounts of data. More and more people started to adopt online interaction with each other and leave digital foot print. The cost of harvesting insights from these interactions fell to marginal low costs. It is not hard to believe when future smart devices (i.e. AR, VR) became popular and affordable, more company will be impacted by not equipped with skill to harvest this ever-growing huge amount of data into useful insights.
The premise of using big data well is to “assume that data is available and not be limited by the amount”, which I have always taken to heart. In the age of Big Data, we need a new way of thinking, and it is far more important than data resources and any algorithms.
I think we are still a long way from the day when artificial intelligence will rule the world. But the winners will always be and should be those who have faith in data because they believe that the purpose of technology is to make people’s lives better and happier. The data network effect provide huge contributions to enhance user’s experience that make Google, Facebook, Amazon, Alibaba beome the most valuable company in the world.
Looking back over the past 20 years, I feel fortunate to have worked for data-enabled pioneers such as Microsoft and eBay. I am even more excited to have been part of a world-renowned data company such as Alibaba. My dream of Big Data is realised along the journey.
After completing my mission, I left Alibaba in 2016 to join Sequoia Capital China as a venture partner, with the initial intention of gaining a broad and deep understanding of the difficulties and opportunities encountered by different players in the digital ecosystem.
As a pleasant surprise, this period coincided with the accelerated promotion of a data-driven digital economy in various cities such as Beijing, Shanghai and the Guangdong-Hong Kong-Macao Greater Bay Area. Therefore, while working as a data strategy and governance consultant for some internet companies with large amounts of data, I was also commissioned by the Chinese Association of Hong Kong and Macao Studies under the Hong Kong and Macao Affairs Office of the State Council to complete the Research Report on “Data-driven Guangdong-Hong Kong-Macao Greater Bay Area Innovative Development Planning Research Report”. In addition, as a consultant to the Beijing Municipal Government’s Big Data Advisory Group, I also wrote the “Use of Big Data for Precision Urban Governance Report”.
What I’ve seen and heard over the past ten years has gradually made me determined to propose a top-level design for data-enabling approach in digital transformation, from strategic thinking to actual implementation. I have always believed that data-enabling approach is an essential factor for the future evolution of business and society and that a top-level design, a practical approach that combines advance technology and human intelligence for the new era is urgently needed on the road to change.
editor remark : Data enabled organisations are those which use their data to deliver their business strategic goals and achieve successful business outcomes either for the company, customers or community across which they operate.
I want to emphasise that in any digital transformation, there are two sides to the data enabling approach (see Figure iii):
1) Incubation process centred on the data-driven application (application propelled by data aggregation-interconnection)
2) Harvesting process centred on the data-growing of relevant data resources (data aggregation-interconnection propelled by application), with the data strategy being the control centre for priorty setting.
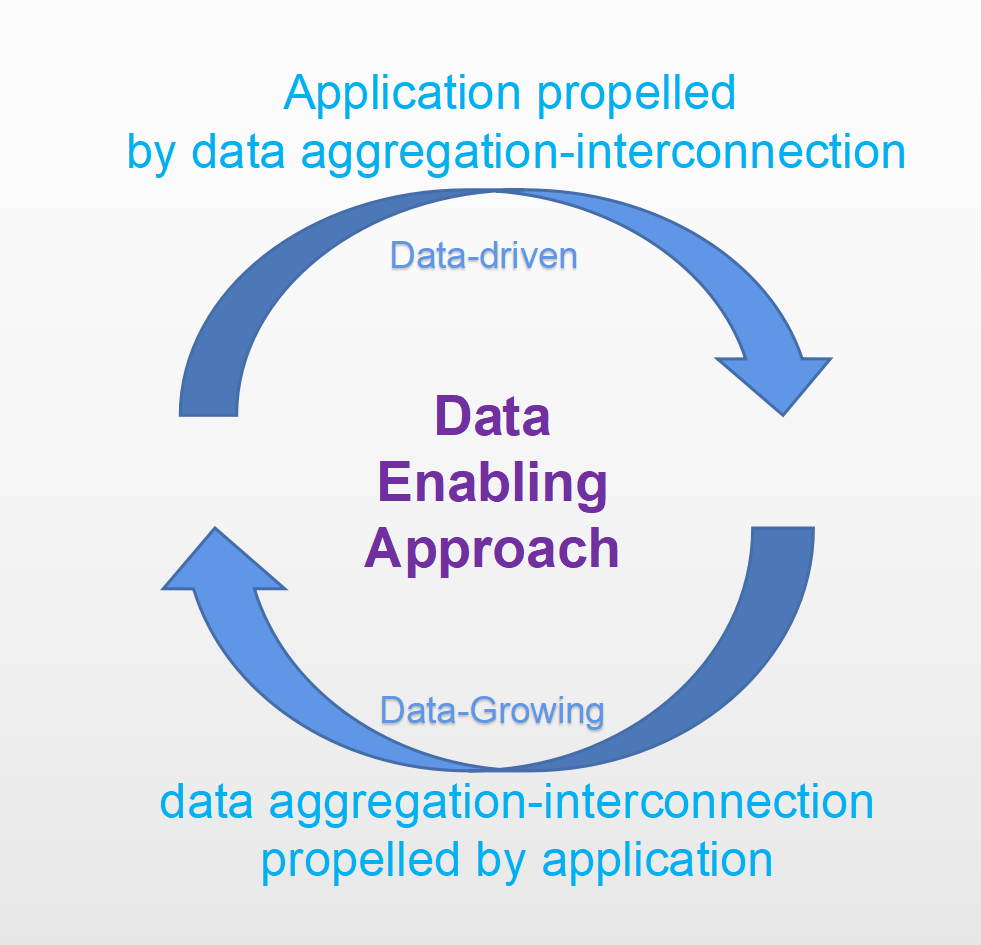
The logic of a data-enabling mindset constantly impacts the inherent way of learning, organisational behavior, and business operations’ underlying logic. Some corporates have compared this change to “changing the engine of a flying plane”. This analogy is not an overstatement, and the impact of this change may go far beyond what we imagine. If the organisational structure of the enterprise does not complete the transition successfully, the whole organisation may be out of control.
With unmanned driving, robots and artificial intelligence, technology has become a means of innovation for many corporates. However, whoever has mastered the various super applications that people use in their daily lives, such as digital maps, eCommerce, search engines, personalised recommendation applications, ride-hailing applications, mobile payment applications, government service applications, credit rating-related applications, etc., will accumulate a large amount of data resources and invariably influence and control various scenarios of people’s lives in the society. It should be noted that digital transformation does merely provide connectivity and better service among user, but also give rise to data network effect. By means for automation and augmentation, AI and super computing power enables learning from the data collected while user leave digital footprint with the applications.
Recently, there has been a lot of unexplained turmoil that is heralding a new era. We are at a tipping point between this old and the new era, and no one can tell us what the future will look like, but having a data-enabled capability is undoubtedly one of the major influencing factors. I predicted in my book “The business Revolution of Big Data” in the year 2013 that data will become a battlefield for countries and corporates in the future. The importance and urgency of this are already self-evident, judging from the cross-border data regulations countries have roll-out from 2016 to 2021.
【Five significant stages for digital transformation in Alibaba group】
Consolidating my Six years of experience in Alibaba and my consulting experience in JD Digital in recent years, I want to explain the connotation of data-enabling approach (both data-driven, data-growing) within digital transformation into five stages:
Stage 1: Using data as the aiming device for decision-making (Data-driven focus)
We first hope to use data as an aiming device to help companies understand the status quo and make favourable decisions for their business model. These decisions include customer acquisition, category management, marketing planning, risk management, and resources allocation ,etc. Informed decision making is one of the building blocks of company success. The goal of data analytics is to enhance decision quality and tranform insights into operational actions. However, as I mentioned in the book “The Nature of Big Data”, it’s not easy to form a good understanding of inter-relationship between “data gathering, generate insight, making decision, action execute” from the perspective of data anlysis in business operations.
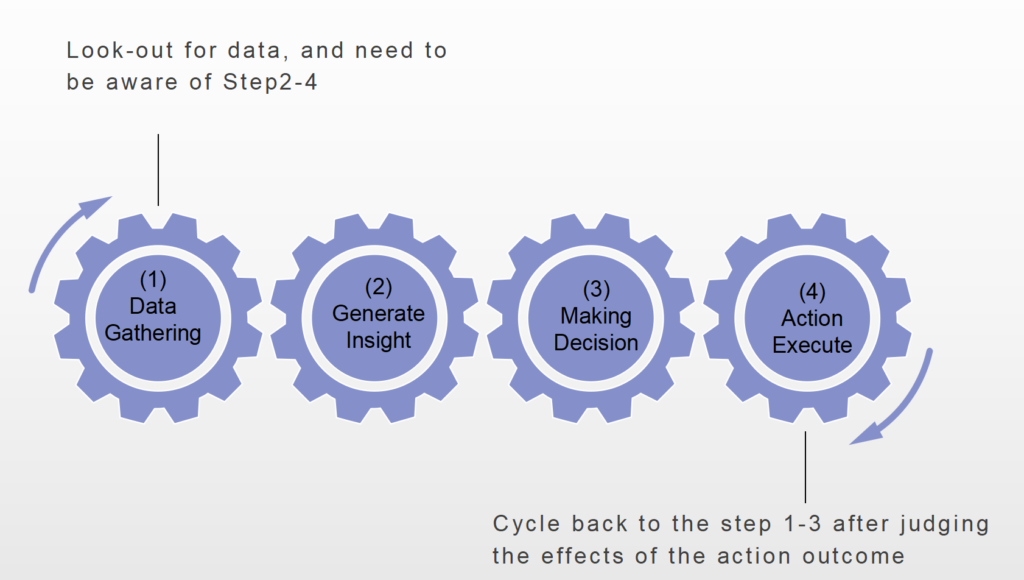
At this stage, we use data analysis to assist human intuition (experience-based assumptions) in making decisions. The realised outcomes of the decision will be evaluated to explore for additional data resources, better contextualised insights, reduce human bias etc., . In this way, we can improve our decision making process, but the problem is that the more ambiguous the answer, the longer the iteration process will take.
To gain experience and make the less digitalised and data oriented decision process traceable, it is essential to be proactive in promoting digitalisation and adding data that has not been collected or utilised to strengthen the metrics, data tracking and visualisation systems. Managers need to lead as role model and make decision-makers at different levels more aware of and capable of making data-driven decisions.
In Alibaba and JD Digital’s monthly management meetings, the CEO often asks executives about the details of data metrics and what they mean for the business. It is only in this pragmatic environment that analysts, business people and product managers can play a “point and shoot” role.
A data-driven monthly management meeting should be like drafting military tactics over the sandbox exercise in war. I would advise you not to be obsessed with fancy big data Screen. A good looking ‘dashboard’ doesn’t always work well.
Stage 2: Embedding data analysis into workflows (Data-driven focus)
I believe that data-driven approach will only work when data capabilities can be generalised to the ‘nerve endings’ of the business, as simply equipping employees with data analytics software and awareness is like scratching the surface; you need to find ways to apply analytics solutions to the decision-making process in the existing workflow or operation.
A typical example would be during a festive sale of an eCommerce company when selecting products or suppliers for the promotion campaign; category managers can use analytics tools that embedded in the existing backend operation platform instead of bouncing around in several systems. Improving the user experience and appreciate the value of analysis is necessary to generalise data analytic capabilities, which is a process of popularising data-driven analysis and a milestone in the digitalisation of the industry.
Another typical case is the analytical capacity within a product developement cycle, where the data that needs to be collected must be thought out and equipped with the relevant data analysis tools when designing the product; otherwise, it is hard to obtain the data to uncover the unfulfilled user demand and trends.
Stage 3: Data governance allows internal and external resources to work seamlessly together (Data-driven + Data-growing)
Ali Finance was Alibaba’s first data innovation business subsidiary in 2011, providing credit services to Small and Medium Enterprises (SME). Its data sources were initially mainly generated from transaction data on Taobao and Tmall, which could be used to assess risks and repayment cycles for lender (bigin with existing seller), etc. As a new business unit within the Group at the time, aggregating data (Data-growing) from multiple sources across companies (later extend to external data source like telco) and maintaining sound data quality on top of that was the biggest challenge for Ali Finance. Data governance play important role to allows this internal and exterrnal data resources to work seamlessly together. (See ch 4-6)
Data quality are parts of the data-growing process that cannot be circumvented, and the greater the span, the greater the difficulty. That is why data integration in large corporation is easily protracted, but the value it brings is very high once interoperability is achieved.
Ali Finance’s (Later became important part of Ant finance) success had set a good example for alibaba management to demonstrate how data network effect take place. Data considered redundant in scenario can become a treasure when integrated under another scenario. Data network effect rise to the extent that the learning is across users. (Seller in this case)
In the data consultancy work I’ve been involved in, the success of this stage directly impacts the probability of success in Stage 4.
Stage 4: Finding the right time to eliminate data silos (Data-growing focus)
It is common to allow each business unit in the same cooperation to use multiple application (i.e. CRm, Marketing, Finance, website ) to operate there own business which largely generate segregation of data.
The more companies using data techonology, the more problem of algorithms, analytics and AI teams working separately in different lines of business, and also because these teams generally each have their data platforms; thus, data silos are common. In this case individual business unit will have limited visibility of hoslistic view of data resources in the company and therefore restrict collaboration among teams. For example in the early stage of data transformation, Taobao and Tmall both have there own recommendation engine with seperate data resources and platform but over 80% of the customer in common. Data analyst on both side found it hard to understand the customer behaviour fully.
Since data silos leads to poor decision making and impact on performance. Many corporates are only beginning to bridge the gap between data silos and integrate data because of the establishment of the Data Management Committee. It was only through the determination of Alibaba’s “Temujin” (Lu Zhaoxi, Alibaba’s Group CEO after Jack ma) to transform the company digitally that I was able to set up Alibaba’s Data Management Committee (See Ch 3). Perhaps because of the success of Alibaba’s digital transformation, “how to build an effective data management committee” has become the question I am asked most often. The answer to this question varies depending on the maturity of the organisation in terms of digitalisation.
In the age of AI and big data, use right data at the right time to generate insights is particular critical. Company need to adopt AI (machine learning) to be data-driven to gain competitive advantage. But one of the biggest barrier to attain success is data integration capability with unstructured or semi-structure data at scale. But the first step to determine success is a ROI question which data silos integration will benefit the most and to create maximun value to the whole cooperation.
Having said that, do data silos have to be eliminated entirely? We will continue to discuss this issue in later chapters (See Ch3-4). In many businesses, it’s a matter of timing to eliminate data silos. For example, if Taobao and Tmall had not integrated their data silos in 2013, it would have been much more challenging to deal with later. But it was not the case when Tmall still at the start up stage in 2009, when the business need to focus to prove the business model.
Stage 5: From business intelligence to intelligence business (Data-driven + Data-growing)
At an Alibaba president’s meeting, Jack Ma once asked: Taobao and Tmall customers are patronised 24 hours a day, so why don’t executives have to work overnight and on weekends? With this in mind, it led to Juhusuan (subsidiary of Taobao) Group Buying’s automation in operation and the emergence of unmanned supermarkets and intelligent customer service.
As the first person to lead a team to develop this automation project, the strength of the data governance and the accuracy of the algorithms I experienced during this process were several notches above the previous four stages. Crucially, you find that data sources you hadn’t even considered collecting before and that many of the decisions you take for granted are uninformed.
The most interesting part of the automation project is that the business, technical, product and data departments all think they are the core departments for operations automation, so the first thing to fix before driving an automation project is the hearts and minds.
On the other hand, decision automation or augmentation of decision making can often provide better decision result than human. However these benefits can be easily undermined by poor quality or missing data during system design. My learning from this stage is that data quality requirement for automation project tend to be higher in term of data governance afford.
The above five stages do not necessarily occur sequentially in a company’s digital transformation process, and the nature of the business varies from company to company. However, the most common mistake is to overlook long-term strategic needs in favour of short-term efficiencies.
Data governance is a long-term capability-building process easily overlooked in many corporates I have met in the digital era. I would therefore suggest that we focus on the following three key questions:
● Question 1: Is there someone in senior management dedicated to look after a data strategy and its execution?
● Question 2: Has the scope of data-driven, data-growing and data governance been aligned and planned in line with the data strategy and prioritised for the long, medium and short term goal of business?
● Question 3: How can technology help reduce manpower in the process so that human resource is no longer a bottleneck in data-driven, data-growing and data governance respectively, including deploying automation to improve the data productivity and efficiency of data use? It is important to note here that different stages of the strategy should use the appropriate management approach and right technology.
I have highlighted the difference between these stages because I have found that many businesses tend to focus on short-term solutions and ignore the systemic problems in data-enabling transformation. Once they reach a stage where data becomes unwieldy, the business needs to grow rapidly, everything becomes purely demand-driven, and the underlying platform set up inevitably lags. Over time, these tasks, which require a great deal of internal coordination, become increasingly difficult until the resources within the organisation are depleted, causing the organisation to lose confidence in its long-term growth. There are three ways in which companies can improve this problem.
● First, try to take an initial review of data-related development projects in your organisation over the past 12 to 24 months. If you find that more than 70% of projects have been prioritised for short-term gain, be wary as this is likely to indicate a lack of focus on long term data governance within the organisation.
● Secondly, look at how data is being shared across the organisation. If you find that the same large data source is being stored repeatedly many times, this means that data usage chaos has festered.
● Finally, many corporates are at odds with data governance and business priorities. In large corporations such as BATJ, some even have more than 3 data platforms with the similar function and replicated data resources. It’s not uncommon to see this phenomenon, but how to deal with this conflict?
Suppose the above description sounds familiar to you and you are suffering from resistance to doing business properly as a result. In that case, my advice to you is that better data strategy is urgent.
BATJ refers to the four major corporations currently at the forefront of digitalisation: Baidu (B), Alibaba (A),Tencent (T) and JingDong (J). –Editor’s note
Extra reading:
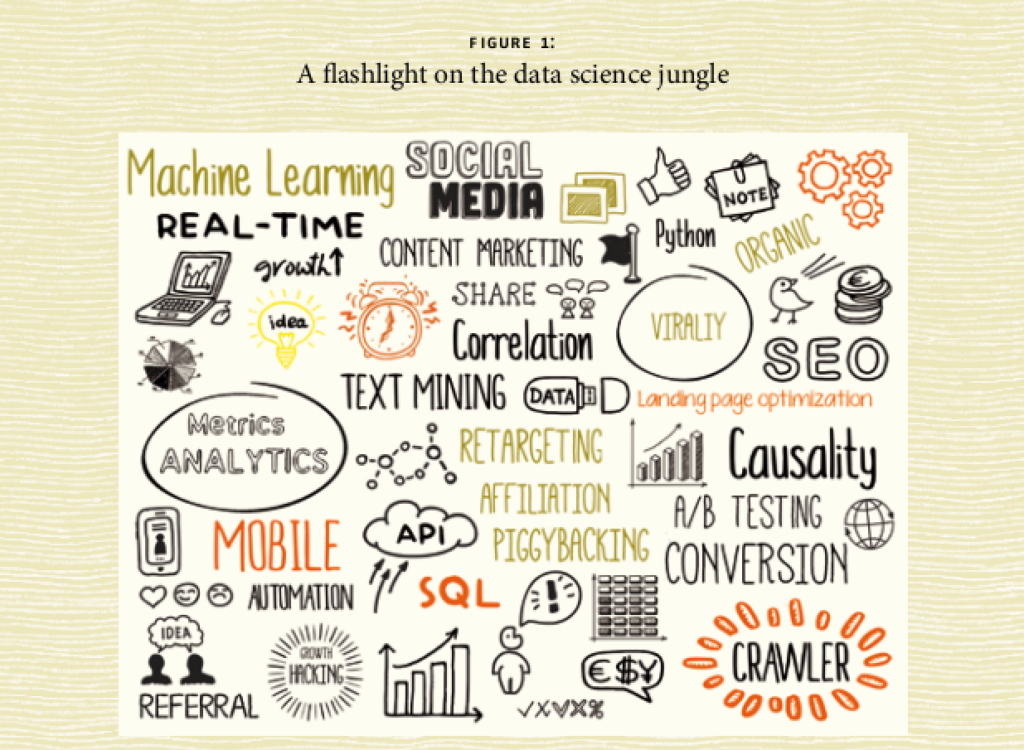